Understanding Text - the next data challenge
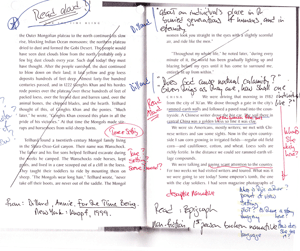
Data Analytics and Natural Language Processing are emerging as potential lifesavers for modern organisations at risk of drowning in rivers of unstructured data. ABBYY is one of a number of leading enterprise software developers to apply artificial intelligence (AI) to the task of transforming the rushing torrent into intelligent and actionable information, via a technology known as Compreno. IDM asked Yury Koryukin, Managing Director of ABBYY Australia, to explain how semantic understanding of natural language can help organisations keep their head above water.
IDM: The ABBYY brand is best known for its OCR and data extraction software. Why has the company sought to develop linguistic technology?
YK: Actually, it’s a logical move for us as ABBYY has spent the past 25 years working in this area to develop the electronic dictionaries used in our OCR products. Over that time, our linguists and developers have created a universal description of language for English and Russian initially with a tree of meaning that accommodates and understands the many synonyms that provide a challenge for search engines. For instance if I am looking for a table is it a collection of data or a wooden thing with four legs? Our technology combines syntactic and semantic analysis, as well as machine learning on untagged text corpora. So, it can resolve various complex language phenomena, including lexical ambiguity, recovering omitted words and links (ellipsis), identifying pronoun referents (anaphora), co-reference, coordination and others. We see a lot of opportunities to use this research to extend the functionality of our current products and introduce entirely new ones.
IDM: ABBYY has recently launched a suite of intelligent capture and language-based analytic solutions: ABBYY Info Extractor, ABBYY Smart Classifier and ABBYY Intelligent Search based on Compreno technology. What exactly is Compreno?
YK: ABBYY Compreno is the result of linguistic research by ABBYY to bring machine analysis a step closer to human text processing. Unlike technologies based on statistic algorithms and rules, which do not actually “know” anything about the language and can therefore only learn from the frequencies and co-occurrences of terms in the text, ABBYY Compreno technology accurately identifies entities, facts and relationships between them — to assist business processes that depend on reliable and granular content analysis. Compreno creates an XML where words and their context are identified, and this information is available for further analysis.
IDM: There are many existing technologies that promise to have solved the challenge of automated classification, what additional value does Compreno bring to this task?
YK: Most classification technologies are based on statistics and rules, and don’t use the actual meaning of words, so if you have a big variety of document types and therefore a high degree of complexity of classification you need to spend quite a lot of time to verify the classification results. Whereas ABBYY Smart Classifier offers innovative language-based classification. It delivers syntactic and semantic analysis of document content to accurately assign these documents into predefined categories. This can be used to automate many processes that require document sorting, routing and archiving. For example, mailroom routing, where it analyses document content and by using meaning-based document attributes automatically routes all kinds of documents to the required people and/or department.
IDM: How does it help Enterprise Search?
YK: On the Internet, Search engines analyse user clicks to improve the quality of search results. These rankings help us to find what we are looking for, although we are still forced to frame our search using keywords instead of natural language. On a corporate network with hundreds or sometimes thousands of users, these ranking methods don’t help. It is not enough to employ statistical methods.
That is why ABBYY’s solution is to generate the results based on ranking by meaning. Unlike traditional ranking, this is built on syntactic and semantic analysis of text, displaying the results that are closest to the queries’ meanings right at the top of the list. It also helps to narrow down the search by selecting the exact meaning for an ambiguous word in the query.
For example, if you search for “software” and “program”, it will understand that you are probably interested in associated terms, and will deliver results for "application" and other suitable synonyms.
"We see many applications of our Compreno technology to exploit the ability to extract “facts” from unstructured documents, for instance entities and relations between them in agreements. There are also opportunities for semantic classification, enterprise search and sentiment analysis." - Yury Koryukin, Managing Director of ABBYY Australia.
IDM: Do you have some examples how these Compreno-based solutions are being employed today?
YK: It is pretty new technology which we just launched a few months ago, although there are already some deployments. One is in the processing of remittance advice documents for a large manufacturer. This organisation supplies products and services to a number of corporate customers (including resellers) who it invoices every month. It then receives large volumes of remittance advices indicating payment was received, however these are completely unstructured and pose a major challenge to capture and process.
It is a complex case as remittance advices can contain different numbers which may not necessarily match the total of a particular invoice. For example, the payment may be only for a half of the bill, or for one and a half, or for the last three bills. As a result, accountants need to manually enter payment details into in their financial system(s).
Traditional data extraction software is effective at handling semi-structured documents like invoices. It’s easy enough to identify and extract an invoice number from an invoice, but it is much more complicated to identify this in a remittance advice, when the format and presentation can vary considerably. It is possible to accomplish this via a rule based approach, but in the case of this manufacturer it assessed the effort to create these rules and to describe exceptions was so large that it made it commercially unreasonable.
Instead they used Compreno and its native ability to identify key entities and extract the interrelated facts. With this technology they were able to identify the invoices for which payments were being made and the actual amount that was attributed to each of these invoices. Thereby significantly minimising and in many cases completely eliminating the need for manual data identification and entry.
IDM: What are some other examples of how this technology could be deployed?
YK: It would be quite possible for ABBYY Info Extractor to be used to help manage the risks presented by having hundreds or thousands of contracts stored in an EDRMS or a fileshare repository.
For example, if there is a contract signed that comes into play in case of a particular event or a circumstance, then how is your organisation alerted when this event takes place? Or if there is a contract signed that guarantees exclusivity to one customer or supplier, and whoever signed this contract leaves the organisation and another contract for exclusivity is signed by someone new, how can the organisation be alerted to the breach? Info Extractor SDK does this though analysis of existing contracts within an organisation to help assess the risks involved. For example, if we have a 400 page contract with lots of conditions, moreover, it is one of many. How can we be sure when entering into another contract of 400 pages, that it does not conflict with the one that is already signed? Currently, this check is performed by legal departments of companies by simply reading the conditions. Compreno can facilitate this work: identify major entities, relationships between them and relevant facts critical to the contract. The decision, however, is still up to the human.
IDM: One of the challenges that usually comes under the heading of Big Data is analysing millions of social media posts, is this an area you are targeting?
YK: We have one global manufacturer already employing Compreno technology to assist with sentiment analysis in social media. It needed to evaluate thousands or more likely millions of online customer reviews on a particular product to provide a score, indicating how well it had been received by the market. Obviously it needed some form of text analysis to do this, but traditional approaches fail in the face of a reviewer who employs irony. For example, “I’ve just purchased the amazing new model of digital camera with some incredible image capture specifications and a promise of remarkable performance, however, unfortunately, the pictures are terrible. In this case a statistical approach would come up with a positive score, a completely inaccurate reading of the review, while Compreno will understand that the total score of the review is negative. We have only really scratched the surface in discovering how our linguistic technology and AI can help in integrating unstructured information into intelligent business processes. Language-based insight into unstructured data will open up new opportunities to action information and improve critical business processes that mitigate risks, increase efficiency and drive revenue.
For further information about ABBYY Compreno technology and the Info Extractor, Smart Classifier and Intelligent Search products, contact sales@abbyy.com.au or phone 02 9004 7401.