A flexible approach to unstructured data
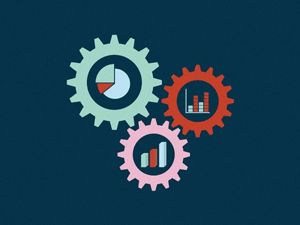
“Data is transforming business!” is a common eureka-esque cry in the anglophone media, as if it’s a new thing. I mean, tell the people of the Neolithic Near East something they don’t already know. Advances in information abstraction, representation, storage, processing and transmission have been transforming industries and whole economies for centuries.
Of course, the irony of that platitude is that it’s also true. Data science is booming: its impact is felt throughout our business and personal lives, and companies are thoroughly aware of the valuable insights that can be gained from systematically analysing data, not to mention the potential dangers of not doing so. “CEOs: Model or Die”, as Bryan Schreier, a partner at Sequoia Capital, wrote in Forbes in July.
Luckily for the CEOs wondering which of modelling or dying would be more enjoyable, it is becoming easier and cheaper to work with data without the need to create intricate clay tokens or access a powerful mainframe. From tiny start-ups to massive multinationals, companies now can get high-quality tools at affordable prices to: ingest, store, manipulate, analyse, or visualize data; to use data to power statistical inference or train and feed machine learning methods; and to route the results of data analytics to their clients or users wherever they are in the world, in near real-time, for those end-users in turn to ingest, store, manipulate and analyse it as they see fit.
There is one annoying limitation, however. For data to flow freely from this pipeline of tools and analysis into valuable models, visualisations and automated reports, it must be structured. It needs to be standardized, organized and computationally searchable. On the face of it, that sounds achievable. After all, there’s enough structured data about — petabyte after petabyte is constantly flowing from banks and cars, credit cards and mobile phones, power grids, websites and electric toothbrushes.
But data is not all equal. If you’re working at Philips on a new addition to the Sonicare range then structured electric toothbrush data is hugely valuable; if you’re not, it’s nothing more than a curiosity. One person’s amusing distraction is another’s golden source. Finding data which is pertinent to the questions you’re asking of it is the first stage of any data analysis project, whether that means images and blogs for insight into the fashion industry, news articles and company press releases for equity markets, or expert opinions on when and where the next hurricane is going to hit the Eastern Seaboard for insurers. The problem you face in these cases is that the data pertinent to your questions is all unstructured, or in the latter case not even necessarily recorded.
And if you’re in that position you’re not alone. Most data is unstructured: as much as 90% of it, according to market intelligence firm IDC. It’s the data of documents, images, audio recordings, videos, blogs, social media posts, and so on. The human heuristics to deal with the inconsistencies, inaccuracies and idiosyncrasies of these types of sources are incredible. We all ingest, store, analyse and use it to make decisions in our heads all the time, in near real-time, and yet they are extremely challenging to deal with computationally.
As a result, most unstructured data — and let’s remember that means most data — remains inaccessible to the models being built by Schreier’s CEO, who is desperately trying to keep up with the herd. Companies are finding immense value, transformative insights, from analysing the 10% of data which is structured, but there is so much more currently locked up in unstructured documents.
So how can companies tap into the opportunity hidden in this data? Well, right now there isn’t a readily available suite of tools for dealing flexibly with unstructured data. It’s too complicated a process for a one-size-fits all unstructured data engine; for anything other than the most trivial data or source it’s a complicated, multi-stage process. Let’s take the example of building a dataset of business relationships from a press release archive and a news feed.
Photo by brotiN biswaS from Pexels
Here are some of the basic steps involved:
· Filtering the corpus for relevant articles
· Identifying people or companies mentioned in the article
· De-duplicating them (when they are inevitably described differently in different parts of the article or between one article and another)
· Establishing which of them are truly involved in a business relationship rather than simply being referred to in passing
· Clustering articles which refer to the same relationship
· Categorising the relationship described by a cluster
· Quantifying the relationships in some way
Some of these steps can be approached computationally, for instance using optical character recognition techniques to produce machine readable text if the original articles are images, named entity recognition to extract company names from the articles, and clustering techniques to group the articles together. Such methods clearly have considerable advantages over the brute force of a purely human effort — saving significant time and money.
Although these methods are powerful they only solve part of the problem, and often with data quality which leaves something to be desired. Their limitations derive from their relative inflexibility in terms of: a) the range of questions that can be posed; b) the subtlety of those questions; and c) the potential for considerable variation between the documents and idiosyncrasies within them. Humans on the other hand demonstrate all the flexibility, intuition and experience required to deal with these problems accurately; after all, press releases and news reports are designed for human rather than computational consumption.
It is, however, true that the balance between what automated methods can achieve and what is more accurately and practically done by humans is shifting rapidly with changes in technology. It also varies from question to question, source to source and business case to business case. This variety in the appropriate solution for a given question — as well as the variety of tasks and questions that can be posed — shows why generic unstructured data tools are thin on the ground.
It is our belief at Hivemind that the most effective method of dealing with a range of unstructured sources is a flexible combination of man and machine.
Our software acts as a workflow tool for data processes, co-ordinating automated and human tasks as appropriate to deal flexibly with both bespoke dataset creation as described above, and practical everyday dataset maintenance. It allows users to break down the task into bite-sized pieces and distribute them to automated or human methods as appropriate.
In many cases that means automated methods to do the heavy lifting with human effort concentrated on cleaning it up and on the more complex steps better suited to human intelligence. This framework is adaptable both to future advances in NLP or broader machine learning and also to the inevitably rich variety of questions and problems that businesses have to ask of their unstructured data.
Daniel Mitchell is Co-founder and CEO of Hivemind, a data science and technology company specialising in the application of human and machine intelligence to complex unstructured data problems.