Lighting up your enterprise data
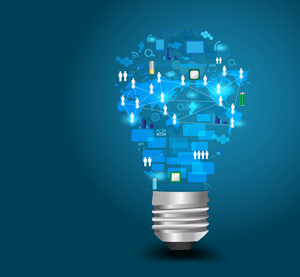
The challenge of automating information extraction is at the core of the Sintelix text analytics platform from Semantic Sciences, a CSIRO spinoff established in 2008 by founder Daniel McMichael and now being utilized by corporate and government customers. in Australia and globally. At the US Department of Defense it is employed in tandem with data analytics technology from tech darling Palantir, best known for its work on behalf of the US government’s intelligence community. Simon Kravis interviewed Daniel to learn more about the commercial uptake of this sophisticated text analytics solution.
SK: What the current state of the art for computers processing text?
DM: Computers have been processing text since the 1950s – so in the sense of simple raw text processing, the tools are well established. Of course the real game is finding new ways to lower costs and create new benefits, products and services. Before we tease those out, let’s pause for a second to think about what presence of written language has done for computing: in some sense, it’s an embarrassment – because representing what a passage of text is saying with digital computers is not easy. But this mismatch has become an amazing opportunity to bring the richness of the concepts and relationships that text can express into practical software.
The biggest productivity gains come from replacing the reading and transcribing documents by people with accurate automatic information extraction. The biggest innovation opportunities derive from novel technologies such as summarisation, the ability to induce networks from data and linking information across documents and databases. These capabilities are progressively revolutionising eDiscovery, investigation, intelligence, research and many other areas.
Let’s look at a basic business process: you load your input data from a file store, record management system or scan it from paper. Then you extract some information from the data, which is then stored, sent downstream or visualised. Processing scanned legacy data obviously requires optical character recognition (OCR) – and while modern day OCR is good enough for search, it makes far too many errors to be useful for information extraction. One of the capabilities Sematic Sciences provides is automatic error correction which greatly reduces OCR error rates. This enables us to tackle many previously impossible information extraction tasks.
Further down the pipeline, beyond OCR, lies information extraction, where there have been significant improvements in generic web scraping and the number of languages offered by entity extractors that find valuable information such as names of people, places, and organisations in unstructured text. The most significant improvements are found in narrow areas with high commercial value, such as extracting data from invoices and resumes.
Overall, I think that there have been significant improvements, but broad coverage high quality solutions are hard to find. We often see that the complexity and range of product offerings can leave customers confused and they often end up with inferior or high cost solutions. I think that there’s a real knowledge gap.
SK: How did Semantic Sciences and its product Sintelix get started?
DM: Semantic Sciences kicked off 6 years ago. We spun out from CSIRO and won a contract with Prime Minister and Cabinet to do a stack of interesting stuff to help the intelligence community in the text analytics area. After about three months, the steering committee called us in and suggested we tear up the statement of work and focus on creating a system for extracting entities (like people, locations, times and events) from free text – but with much greater accuracy than was available on the market. It was a challenging task – but we were happy to oblige, especially when after 18 months we had brought Sintelix to life and created a solution with on fifth of the normal error rate. The success of our company owes a great deal to their courage. Our entity extraction capability remains world best.
Since then, we’ve created a raft of new text analytic capabilities: network creation, network exploration and decision making. These have opened the way for us to create tools for fraud detection, metadata extraction, predicting group decisions, financial analysis, records management, recruitment, intelligence and defence. Besides accuracy, we have focussed on providing a world-leading suite of configuration and tuning tools, so that users can gain outstanding results for their tasks on their data. For example, we recently did a project to extract metadata from archival patents so that they can be effectively searched. The agency involved seemed amazed that it was possible to achieve the quality we provided. There’s little human intervention, so it’s also very cost-effective.
A recent development has taken us into analysing group decision making process for an issue. Sintelix gathers information from across large numbers of documents to create a table of stakeholders and their key parameters. This task came out of work we are doing for the US Department of Defence. Topic analysis is the first pass; the second identifies stakeholders. Sintelix then determines the stakeholders’ positions on the issue, how much they care about it and how much influence they have relative to the other stakeholders. We’ve helped them reduce the effort for this task from about a month to half a day, which provides them with good and timely initial intelligence estimates.
SK: How does Semantic Sciences work with Palantir? (Backed by the CIA and US National security Agency, Palantir is a Silicon Valley startup now valued at over $US9 billion that grew out of efforts by PayPal co-founder Peter Thiel and Stanford engineers who wanted to track down Russian crime syndicates that were defrauding the payment company.)
DM: Palantir is a widely used data analytics tool that works very well on structured data. It can ingest documents, but documents have to be manually marked up to identify entities to be processed. This is a very slow process. The Sintelix plug-in for Palantir can identify entities automatically and then link them. This greatly improves the productivity of Palantir installations which work with documents.
SK: Do you think text analysis will be a part of mainstream computing in future?
DM: I think that this process is well underway. Obviously, the lead industries cluster around the internet, but benefits to productivity and effectiveness from quality text analytics are already having a huge impact in many C2B, B2B and internal business processes. I think the development of the text analytics market is following the classic profile of supplier proliferation, then progressive incorporation into main stream products and finally consolidation. We’re currently experiencing both supplier proliferation and mainstream incorporation.
SK: What plans does Semantic Sciences have to avoid a consolidation squeeze?
DM: We’re fortunate in having a lot of high quality, well integrated, easily configurable technology. That’s helpful, but doesn’t provide any guarantees. One of our key strategies is complementing well-known mainstream products including Palantir, I2, SharePoint and SQL-based DBMSs. We also focus on international markets, initially the US, then Canada and now the UK. Our technology is being “white label” incorporated into main stream products. Even though we have strategised for growth, the life blood of Semantic Sciences is technical excellence and making customers happy. That’s what carries us forward.
An award-wining scholar of St. John’s College, Daniel graduated from Oxford University with a PhD in Engineering Science in 1983. His career took him to CSIRO, where he led the team that created the Cognizant Control Room, a research platform developed in association with Boeing as part of Project Wedgetail, Australia’s Airborne Early Warning and Control aircraft. This aimed to provide alerts to an operator’s awareness from his speech and GUI interactions. That solution borrowed ideas from natural language processing - which from the early 2000s became his passion. In 2008, he stepped out of a research career to found Semantic Sciences with a mission to create tools for analysing unstructured data at a new level of accuracy and integration. Alongside a team of researchers he has brought to life Sintelix.
Simon Kravis has worked on tools to deal with it with minimal user disruption at KAZ and Fujitsu before starting his own company, Aleka Consulting in 2013.