The Toxic Positivity of AI
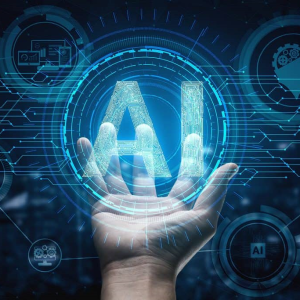
Much has been said and written in recent years about Artificial Intelligence (AI) and its likely impact on the world. So much in fact that, like every technology trend before it, the hype overwhelms the substance. Toxic positivity though? That seems a little harsh given that every modern business aspires to be data-driven in both strategy and execution.
Over the last decade or two, data has been universally adored as a critical corporate asset, essential to quality decision-making. AI’s meteoric rise is therefore no surprise – but is it justified?
All of that said, the genuine importance of data as an asset has resulted in significant overheads being required for its protection and management. Whether we’re talking about security, regulatory obligations, or simply data integrity, it’s clear that there are plenty of risks and concerns about how it is being used.
Recently, the concept of data as a liability has also raised its head, albeit usually in terms of its strategic impact, and what might happen if it was compromised in some way. The prevailing analogy has changed from “data is the new oil” to “data is like uranium”, both powerful and dangerous. Savvy data practitioners now realise that governance, while never sexy, has taken on a new and heightened importance.
Yet that’s not quite what we’re talking about. For me, the idea of toxic positivity being applied to AI, takes two forms – context and presentation. If the broader concept of toxic positivity is a social construct that appeals to popular culture and the zeitgeist of today – why wouldn’t it pertain to data?
In terms of context, it’s easy to see how data practitioners become infatuated with their analyses and reports, and are blinded to more mundane considerations like relevance, and impact. This type of toxicity stems from the idea that data is the sole, and unquestionable (objective) truth.
Overconfidence in your data and algorithms breeds unwarranted certainty around any insights and can yield fatally flawed decisions. The solution to this problem is to maintain a healthy scepticism towards prima facie answers and to apply common sense and experience in equal measure. As always, data should be used to prove or disprove hypotheses, not the other way around.
A more insidious threat to decision-making integrity has emerged though, in the form of Generative AI “solutions”, and more specifically their user interfaces. Obvious challenges with AI include a lack explainability, poor transparency, and variable data quality, however a “positivity” problem now presents itself when we Generative AI’s outputs – delivered in such a prescriptive and authoritative manner, as to silence any debate on their value or correctness.
Here is where AI tends towards positive toxicity – attractive, easy answers that are presented as compelling and “right” answers are the default option for time-poor analysts or inattentive insight consumers. Without any way of knowing if answers are right or wrong, users will naturally opt for the path of least resistance. Unfortunately, once headed down this path, it is very hard for them to turn back.
How then, as data practitioners and businesspeople, can we combat these toxic effects? Luckily, the fundamentals of data quality management always apply and in AI’s case are more important than ever.
Firstly, increased attention to data cleaning and preprocessing can have dramatic effects on AI model quality, reducing errors and filtering noisy data. Ensuring data is consistent and balanced improves model accuracy and speeds computational efficiency (currently a critical consideration for AI). Likewise, preprocessing supports more focused feature engineering, smaller data sets, and reduced dimensionality – all of which means “better” and more relevant models.
Similarly, when training AI models, considerations like data labelling and annotation provide benefits in terms of model validation and generalised applications. Consistent data classification provides a learning baseline, enables pattern recognition/generalisation, and supports data portability across different models.
Furthermore, expert annotations facilitate custom models and/or domain-specific applications – fundamental to the broader adoption of AI.
Like all emerging technologies, Generative AI is battling its way through the hype cycle, with sustained value on the foreseeable (but not guaranteed) horizon. Meanwhile it’s important to remember that whether we’re talking about AI or “traditional” analytics the fundamentals of data quality and integrity always hold value.
There is never any drawback to actually knowing your business, and understanding how data can improve it – this only comes with experience and true domain expertise.
The assertions above aren’t intended to question the value of AI, or data-driven decision-making for that matter. The right knowledge, thoughtfully applied, can illuminate a decision with new possibilities. Rather, it’s to highlight that the fundamentals of data management always apply, no matter what analytical techniques are being applied.
Toxic positivity comes in the form of the attractive soapbox spruiker standing on the corner, telling you they have all the beautiful answers, without getting you to do the work.
Brad Kasell is the Principal Technology Strategist at Domo Asia-Pacific, based in Melbourne, Australia. Brad brings over 27 years of experience in software engineering, management consulting, sales, digital strategy and data protection, and has held previous roles at multinationals including McKinsey, Deloitte Digital, PwC, Oracle, IBM, Microsoft, and Salesforce in Australia and the United States.