Generative AI Mitigating Data Security and Privacy Risks
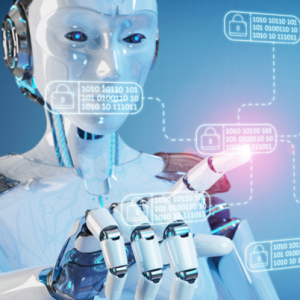
Over the past few months, generative AI has taken the technology world by storm. As per CB Insights, 2022 was a record year for investment in generative AI start-ups, with equity funding topping $US2.6 billion across 110 deals. Whether it is content creation with Jasper.ai, image creation with Midjourney, or text processing with Azure OpenAI services, there is a generative AI foundation model to boost various aspects of your business.
Whether you decide to train your proprietary foundation model or fine tune and prompt tune an open source/commercial foundation model, or a domain specific foundation model as an ISV solution, it is critical that you take the necessary steps to mitigate potential data security and privacy risks.
Some of the commonly asked questions are:
- Can we provide sensitive information to Large Language Models (LLM) either through fine-tuning or prompt augmentation?
- Will LLMs reveal my information?
A common concern is that an LLM might ‘learn’ from your prompts and offer that information to others who query for related things or be used to further train the LLM. Or the data that you share via queries is stored online and may be hacked, leaked, or more likely, accidentally made publicly accessible.
It is critical that you take the necessary steps to mitigate potential data security and privacy risks.
Types of Data
Let us first delve into the types of data a generative AI service or application provider may process if you decide to fine tune and prompt tune an existing foundation model, using the example reference diagram below:
Foundation Models: Approaches and Impacts on an Enterprise Application/Solution
Checklist for Mitigating Risk with Generative AI
Now that you have a baseline understanding of how your data is processed, you need to review how your data is retained and what custom controls are available to you.
Basically, you need to understand how your data sharing as part of fine-tuning or prompt augmentation is managed. To aid with this, here is the checklist of questions you need to ask the provider and ensure adherence to your corporate policies to mitigate your data security and privacy risks:
Does the provider support your ability to opt-in/opt out of including your data for training their model?
- Subject to your use case and for proprietary data, make sure you opt out or, at a minimum, that the training data provided by you is only used to fine-tune your model and not used by the provider to train or improve any of their models.
Can you delete your training and validation data and your fine-tuned models?
- Make sure you can.
Does the provider process the data [prompts, completions, and generated result] to train, retain or improve their models?
- Subject to your use case and for proprietary data, make sure you opt out, or by default the output data is not used by the provider to train or improve any of their models.
- Do not submit any private and/or proprietary data to any public LLM as prompts.
Are the prompts and completions data stored temporarily? If “yes”, how long is the data stored for?
- Make sure it is stored securely in the same region you operate from and is logically isolated with your subscription and API credentials.
- Make sure it is no longer than “N” number of days that is aligned with your corporate policies.
- Make sure it is encrypted, at best, by providers’ managed keys.
Is the data shared with partners?
- Many providers anonymize the data while sharing it with partners. Make sure you are fine with this specific to your use case, this may not be enough for your corporation.
Who has access to it from the provider?
- Make sure only the authorized employees have access to it.
How is the data used by the provider?
- It may be used for debugging purposes in the event of a failure and/or investigating patterns of abuse or misuse.
- The content filtering models are run on both the prompt inputs as well as the generated completions.
Can you opt out of content filtering and logging?
- If your use case involves the processing of sensitive, highly confidential, or legally regulated input data but the likelihood of harmful outputs and/or misuse is low, check with your provider if you can opt out of content filtering and logging.
- Once the provider approves the opt out, make sure they do not store any prompts and completions associated with the approved subscription for which abuse monitoring is configured off. In this case, because no prompts and completions are stored at rest, no provider employees have access to your data, even for limited time.
Does the provider log model usage and support traceability for your compliance needs?
- Make sure they do.
If your use case necessitates the creation of a proprietary model, which means you train your own model, you could either train the model in-house or partner with the model provider that supports training a new proprietary model.
If partnering with the model provider, in addition to opting for VPC for training and hosting the model, make sure you follow the above noted checklist where relevant and ensure adherence to your corporate policies to mitigate your data privacy and security risks.
Ritu Jyoti is Group Vice President, Worldwide Artificial Intelligence and Automation Research at IDC.