5 Reasons Why More Companies Don't Have Data Quality Processes In Place?
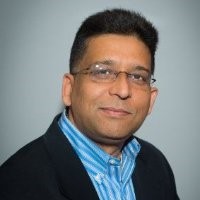
Numerous studies on data management have concluded that poor quality data has a significant impact on an organisation's bottom line (see Bad Data Is Costing the U.S. At Least 6% of Its GDP). However, this doesn't seem to resonate with many organisations and they continue to ignore this issue.
Recently, the CEO of a technology company posed the following question to me - "Why don't more companies have data quality processes in place?". I found this rather intriguing, since it indicated to me that he was actually thinking about this important topic and was eager to get his question answered.
Here's what I told him - "The simple answer to your question is that putting data quality processes in place at any company is very hard". I then shared the following 5 points, to back up my statement:
- Quality is a nebulous term: As I've mentioned in a previous article on this topic "Quality is in the eye of the beholder". By this I mean each consumer of a piece of data or a data set has his or her definition and expectation of quality and they vary quite a bit (the term for this in data management parlance is "fit for use"). How does one reconcile this situation to meet everyone's expectation?
- How does one define the requirements: It is very hard to define data quality requirements for a broad spectrum of data types and data sets. I've typically advised clients to analyse their data quality patterns and use cases and then identify various quality dimensions they wish to measure, to determine the quality of their data. However, there is no agreement within the data management community on these dimensions and their definitions.
- Supply Chain Challenge: If one considers this a supply chain problem (e.g. poor quality data across the information supply chain potentially causes bottlenecks or impacts business processes), then you can see how it will manifest itself within organisations. The challenge is that in order to identify the information supply chain bottlenecks and fix them, there needs to be a clear understanding of the data lineage and legitimate data transformations that have occurred as the data travels through the supply chain. This isn't readily available nor easily done.
- Data semantics is another challenge: In many organisations associates can't agree on the definitions and meaning of data elements and their uses. How can one define the quality requirements, allowable values and other such important criteria if there is a disconnect at the semantic level?
- Immature Governance: Data Governance (DG) maturity at many organisations is either very low or the DG organisation isn't very effective. This results in a lack of accountability and proper mechanisms to address data related issues such as quality and semantics.
Here are some solutions to the above challenges (assuming your data quality program isn't very mature) -
To address points 1 and 2 above identify primary uses of enterprise critical data, who their consumers are and what their quality expectations are. Then articulate data quality dimensions pertinent to your quality use cases and how you will measure them (e.g. tool, code, etc.)
For point 3, develop a crowd-sourced mechanism to identify bottlenecks in the Information Supply Chain caused by poor quality data. You can certainly use existing issue management system for this or build a simple form that is accessible to all organizations.
For point 4, capture semantics related to enterprise critical data in a central repository that is accessible to all. Your governance team should drive consensus and alignment across stakeholders.
If you have a governance organization, ensure that it has the support from senior management and the necessary political clout to improve data management across the firm.
The points I made are based on real life experience gained leading the Enterprise Data Quality program at the largest financial services company in the world. In order to overcome the data quality problems across "Small" and "Big" data, the above areas have to be addressed. They make logical sense, but executing them across numerous organizations that have competing priorities poses challenges. Requires leadership and sponsorship at the C-level and strong data management expertise.
I think he got the message!
Jay Zaidi is an entrepreneur and author in strategic data management. His book called “Data-driven Leaders Always Win“ is available on Amazon. Jay is one of the overseas keynote speakers at inForum 2016, the annual conference of Records and Information Management Professionals Australasia, September 11 -14 2016, Crown Perth.