Four data quality steps you must take for AI success
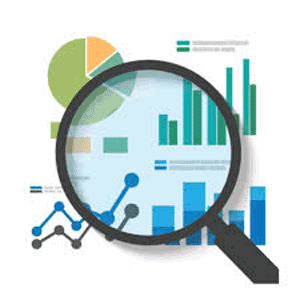
I’m going to go out on a limb and assume your organization is like most others when it comes to AI: you know what it is, you believe in its promise and you’re eager to see what it can do for your business.
A recent Frost & Sullivan survey of 1,636 IT decision makers around the world confirms that assumption. We found that 63 percent of companies use AI and machine learning today, and 72 percent plan to up their investment over the next two years.*
There’s just one problem — most companies won’t achieve desired outcomes from AI if their data quality isn’t, well, quality. And, chances are, it isn’t.
Why AI craves good data
At its core, AI uses advanced algorithms and machine learning to better capture, process and act on information. Whether you’re using it in the contact centre to improve customer and agent experience, on the production floor to optimize productivity and streamline your supply chain, or in the back office to speed decision making and drive innovation, AI needs good information, like our bodies need good calories, to operate at optimal levels.
Why most data quality is a hot mess
Most companies have had years, even decades, to develop a strong and secure data management system — for structured data. Now there are vast streams of unstructured data and terabytes of data that could be used by structured systems entering the organization from disparate sources like beacons, thermostats, smart cars and wearable tech.
Things get messy fast, and the mess grows exponentially nasty — a hoarder’s dream, but a business nightmare. No wonder IT managers say the sheer volume of data is one of the biggest threats to their AI initiatives.
What you can do about it
There are four data quality steps you can take to increase your chances for AI success:
- Treat structured and unstructured data equally. AI is most useful when it can analyse a wide range of information — including text, audio and video — from a wide range of sources. But it’s also important to consider all the structured data coming into the enterprise, including inputs from sensors, beacons and the like.
- Eliminate the noise. This is one of the hardest, and most critical, elements of a modern data management system: determining what information is valuable, and what is flotsam turned up in the tides. Not all information is worth collecting, analysing or storing. Make sure the data you’re gathering serves an identified and prioritized business purpose — and put in place metrics for measuring success.
- Pay close attention to privacy and compliance. If your AI system is scooping up data from a range of public and proprietary sources, some of that information might be subject to specific rules and regulations that don’t apply to the data you normally collect.
- Ensure everyone and every system has access to the data it needs, when it needs it. Information can serve multiple purposes. For instance, knowing that a customer is unhappy with a product serves that specific CX interaction, but it can also feed into product development, channel strategies and more. Take advantage of the advanced analytics AI offers across the organization by surfacing relevant data wherever it makes sense.
How all this data plays out for most companies today
Let’s take one scenario — using AI in the contact centre to improve outcomes — and see how it plays out.
One of the biggest frustrations for many customers is explaining the same problem over again, every time they switch channels. They go to your website for help and see nothing in the FAQ. They launch a chat session, then send a follow-up email and finally make a phone call. They expect, or desperately want, your agent and organization to know they took those actions.
Easy, right? Your modern contact centre software can offer an omnichannel experience that links all those interactions to the same customer for personalized service. Except, how does that system handle the email and phone call?
That data is unstructured. You need to tag it, classify it and contextualize it — in realtime, while the customer waits for an answer. That involves everything from basic translation and voice recognition to advanced analytics that can contextualise key words and phrases for the customer- and agent-side of the conversation.
Once that’s done, the system needs to store the data for future use, and then figure out how to surface it for any advanced data mining you might do to continue to improve processes.
And what if the customer reached out on Twitter or LinkedIn? What if the customer finally got exasperated and went to one of your physical stores to speak with a human? Chances are, those interactions are missed. (And, chances are, that customer is already lost to a competitor.)
Now consider the information your organisation captures from sensors and beacons — from smart badges to wearable tech, building systems to biofeedback. Even if much of that information is structured, it’s often difficult to know where to put it and how to use it — not to mention where it fits in the overall security posture that requires stringent privacy and control over some (but not all!) information.
Exhausted? Overwhelmed? Absolutely. Fortunately, vendors are developing ways to handle this information overload, from vetting data at its source to applying algorithms that can improve Extract-Transform-Load (ETL) processes. But it’s still incumbent on you, your company, to take the necessary steps — and invest in the necessary technology — to ensure your data quality is ready for AI, or don’t bother.
* Source: Top End User Priorities in Digital Transformation, Global, 2019. To access go to frost.com
Melanie Turek is Vice President, Research, Frost & Sullivan. Originally published HERE