Building a privacy layer for AI
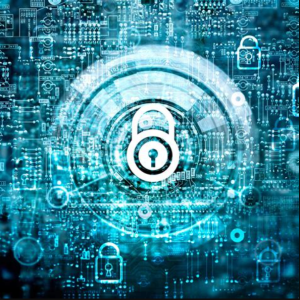
As the democratization of artificial intelligence continues, a new reality is dawning for brands. Data governance is not only a hot topic — it is a potentially lethal pitfall. Brands that want to survive and thrive in this new environment need to bring their data governance policies up to speed. And fast.
How data practitioners think about the confluence of AI and data — how AI models are trained, how data quality is ensured, how permissions are managed — can seem overwhelming. On the ground, many data engineers, business leaders, and sales and marketing teams are looking for an AI data governance north star. What does data governance mean in the realm of AI, practically, as it pertains to privacy and AI?
Regulations are catching up, as seen in 2023 with both the EU AI Act and, in the U.S., the Biden-Harris administration's Executive Order on the Safe, Secure, and Trustworthy Development and Use of Artificial Intelligence.
However, beyond the realm of regulation, there is a pressing need for practical guidance at the grassroots level. So, what steps should teams take to build a comprehensive AI governance framework? The answer lies in proactive engagement and the development of an enterprise privacy layer for AI, which can be broken down into several actionable components.
Defining AI governance
AI governance is a dual pillar system that includes data governance and model governance.
Data governance is relatively familiar terrain for many brands. It involves ensuring data collection, processing and utilization align with privacy policies, user commitments and regulatory requirements. Remember, this extends to employee interactions with AI tools and their usage must fit within a company's data governance framework.
Model governance, on the other hand, focuses on ensuring that both the input, the data used for training models, and output, the data produced by models, adhere to relevant regulations and organizational commitments.
Data governance: A closer look
Data governance can be further broken down into two subcomponents: data cataloging and AI-specific data governance.
Many organizations already engage in data cataloging, maintaining comprehensive data maps and lists of systems where data is stored and processed. This includes tracking data purposes and regulatory compliance, as well as performing data processing impact assessments.
AI-specific data governance involves additional considerations unique to AI applications. Brands must adapt data governance strategies to account for AI's specific needs and ethical implications.
Purpose-built AI governance capabilities
If a specific dataset is used to train an AI model, it's crucial for AI practitioners to determine whether the data purposes are disclosed to the end user. Is their consent obtained for building AI models from their data?
The first pillar of AI governance, specifically, is purpose and permission. This involves clearly defining the reasons for using data, disclosing this usage and always obtaining user consent.
Access control in AI
Access control in AI governance focuses on the data used to build models. For instance, if a model is trained on user data containing unique identifiers, like email addresses, it is worth considering whether such sensitive information is necessary for the model. Often, tokenization or pseudonymization techniques, like hashing with salting, can be employed to enhance privacy. Why use personal identifiable information, such as an email address, when a unique identifier, like simply a number, could suffice? More advanced techniques like differential privacy can also be used to add noise to training data while still allowing models to learn, make accurate predictions and/or make relevant business decisions.
We are already learning from real-world examples that if a user prompts a large language model with enough innocuous information, it can spit out the data used to train itself. If a model inputs personal identifiable information for whatever reason, that data at risk of exposure. Brands must consider why they are inputting data in the first place.
Data quality
Beyond cataloguing, data governance for AI also includes ensuring data quality. This involves checking for bias, representation, labels and feature quality in the data before it is used in models.
Model governance
When building out model AI governance within your organization, consider these three areas:
Purpose and permission: Similar to data governance, this ensures model training complies with your privacy policies and user consent.
Model-data lineage: This is crucial. Model-data lineage is the process of documenting the connection between datasets and models. When deployed effectively, model-data lineage enables brands to look holistically at what datasets are used to train their models and how they compare and relate to each other. This means brands can be confident the data used to train their models is representative of the data that will be used when the model is conducting inference.
Model evaluation and assessments: Finally, it is time to wrap a bow around all this work. Using evaluation and assessments, brands can actively demonstrate that they are doing exactly what they said they would. With the right model evaluations and assessments, they can determine whether a model is performing as intended, what datasets were used to evaluate the model, the properties of those datasets and whether the system contains any bias. Outputs must be fair to the constituents the model serves.
Going one step further, to really build a responsible privacy layer, disturb existing datasets or generate synthetic datasets that have bias, then evaluate models against those sets to verify the model outputs are resilient to bias when the models are used for inference after training.
All this information can be used to prove to the leadership team, business and even regulators, that the company is doing the right thing. Using data governance and model governance for AI is responsible AI governance — from the ground up.
As AI continues to redefine the landscape of data and technology, the onus is on practitioners, leaders and innovators to pave the way for an AI-driven future that upholds the highest standards of privacy, security and ethical practice.
Start with building out data governance and model governance for AI. Think of this as a call to action to embrace the role as a steward of responsible data practices in the AI era.
Let's champion a future where AI benefits all, guided by the principles of responsibility and trust.
Vivek Vaidya is a contributor to the International Association of Privacy Professionals (IAPP) . Originally published HERE