LLMs and RAG ushering the Next Era of IDP
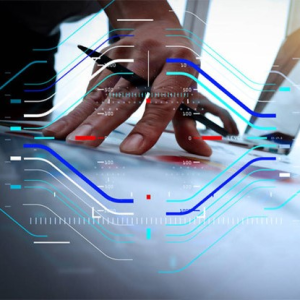
Document automation has become a key tool for enterprises to improve efficiency and reduce costs. With the rise of generative AI in late 2022, Large Language Models (LLMs) like GPT, Gemini and Llama have demonstrated immense potential in document automation processing.
These models haven't simply transformed data processing methods; they've completely reshaped the way documents are processed, enhancing both efficiency and accuracy, particularly in Intelligent Document Processing (IDP).
Now a new approach is on the rise that blends real-world knowledge with LLM capabilities to enhance the ways back-office operations are automated – Retrieval Augmented Generation (RAG)
What is RAG and how does it work with LLMs?
Imagine you have a huge library of books, and you need to write a report on a specific topic. RAG is the super-smart helper who can do two things:
- Retrieve: It quickly searches through all the books in the library and finds the most important information about your topic. It's like having a super-fast reader who can pick out just the right facts you need.
- Generate: Using this information, it creates a report in its own words. It doesn't just copy from the books but understands the information and explains it in a way that makes sense for your use case.
So, RAG is like combining a super-fast library searcher with a brilliant writer. It helps create new information by first finding the right facts and then putting them together in a helpful way.
It feels like using an LLM because it generates texts on domain-specific topics – as a domain-trained LLM would. However, unlike standard LLMs, which are limited to the knowledge they were trained on, RAG goes a step further. It utilizes retrieval mechanisms to access and incorporate outside knowledge, delivering more appropriate and precise information. This is especially helpful for tasks requiring extremely specific or up-to-date information.
RAG, in short, is what truly puts the "self-learning" capability on the LLM table.
Can I use an LLM without RAG and still achieve accuracy and efficiency?
On their journey to intelligent document automation, organizations combine the power of LLMs with domain-specific knowledge, thus deploying the so-called Customized Language Models for standalone use.
Whether they're named Specialized, Customized, Proprietary, or Private, they all refer to the same thing – a domain-specific language model, smaller than an LLM, that excels at handling private data sets within a specific topic.
Despite their edge of expertise, customized LLMs bring some challenges to organizations which slow down or block end-to-end automation:
- Cost: GPU costs for training language models can be quite high, and maintaining a certain number of continuously running GPUs to meet fast response demands further elevates costs.
- Scalability and versatility: Building and maintaining such models demands substantial initial development and ongoing maintenance, combined with very close collaboration across departments in the long term.
- Transparency and explainability: When errors arise or adjustments are needed, pinpointing the issue can be difficult for both customers and technical teams, because LLMs, and especially customized ones, only provide the action and not the reasoning behind it.
So, the answer to the question above is yes, you can. In fact, many organizations do just that, but if they truly want to make the leap towards autonomous back-office operations, they need something extra...
RAG + LLMs: The power combo for document intelligence and automation
Enhancing LLMs with RAG makes it possible to overcome their built-in knowledge limits, produce more informed and contextually rich content, and pave the way for a new era where AI-generated text is not just more accurate, but also more nuanced and tailored to specific needs.
This spills over into many operational excellence gains for organisations:
- Data Processing and Cost-Effectiveness: The RAG + LLMs combination reduces dependence on expensive hardware by optimizing data organization and prompting processes. The versatility of LLMs allows for faster adaptation to different customer needs, reducing the need for customized development and further minimizing cost and time investments.
- Efficient Processing of Unstructured Documents: Offering superior parsing capabilities, image and text data understanding, multimodal LLMs deliver more accurate information extraction and data classification, critical functionalities in the IDP domain.
Traditional processing methods struggle with unstructured documents like complex invoices. Multimodal LLMs, however, demonstrate their strengths in handling such tasks.
For example, in invoice processing, multimodal models can directly analyse image content and combine it with text information to effectively identify and interpret line items and table data without the need for cumbersome pre-processing steps.
- Enhanced Transparency and Explainability: By showcasing the entire decision-making process and reasoning chain, customers can better understand how the model functions and the basis for its decisions, thereby improving user experience and customer trust.
For instance, when an RAG model assigns an invoice to a specific general ledger account, it not only displays the outcome but also summarizes the reasoning behind the classification, such as pointing out the specific document content and historical data patterns that led to the decision.
This capability significantly enhances the transparency of the entire process, allowing customers to understand the logic and rationale behind each decision.
- Provide a better continual learning experience:
The ability to quickly provide feedback on the model allows customers to see improvements instantly, unlike conventional model training methods that require more time, effort, and cost.
As you can see the potential of combining LLMs and RAG extends beyond traditional document processing tasks. As technology advances, new application scenarios continue to emerge, such as automating complex business processes, enhancing customer interaction, and providing real-time business insights.
The bottom line? At Hypatos we are confident that the future of intelligent document processing is all about teaming up LLMs with RAG. The former have already shaken things up in document processing, but the latter is what will take things to new heights if you are looking for spot-on accuracy, cost efficiency, handling complex and unstructured documents, and learning on the fly.
Dr. He Zhang is CTO at Hypatos. Originally published HERE